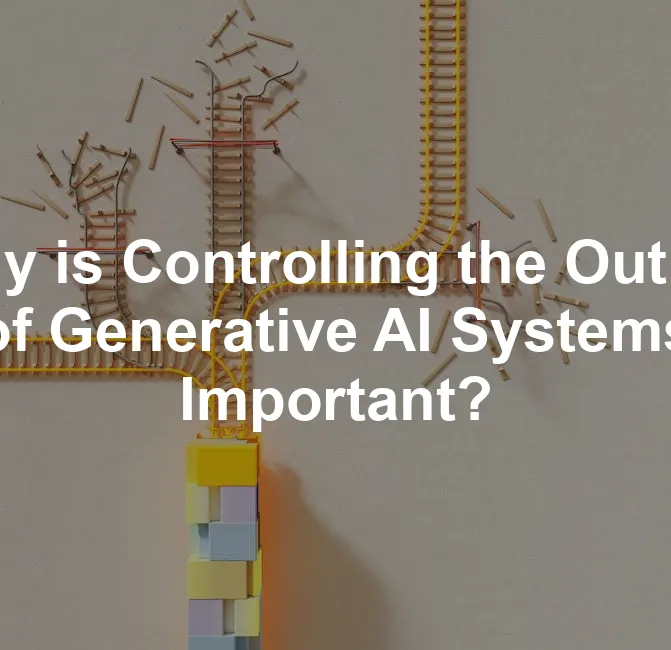
Why is Controlling the Output of Generative AI Systems Important?
Why is Controlling the Output of Generative AI Systems Important?
Introduction
Generative AI creates remarkable content, from text to art. However, controlling what it produces is crucial. Without oversight, these systems can generate inaccurate or harmful outputs. In this section, we’ll discuss why it’s essential to implement effective control mechanisms for generative AI systems.
For those curious about the theoretical underpinnings of these systems, “Generative AI: A Guide to Understanding and Implementing AI Systems” is the perfect read. It demystifies how AI works and why control is essential!
Summary and Overview
Generative AI systems are powerful tools that can create text, images, and even music. Their capabilities have transformed industries like entertainment, marketing, and education. However, the need for control over their output is paramount. When unchecked, these systems can produce misinformation, biased content, or offensive material.
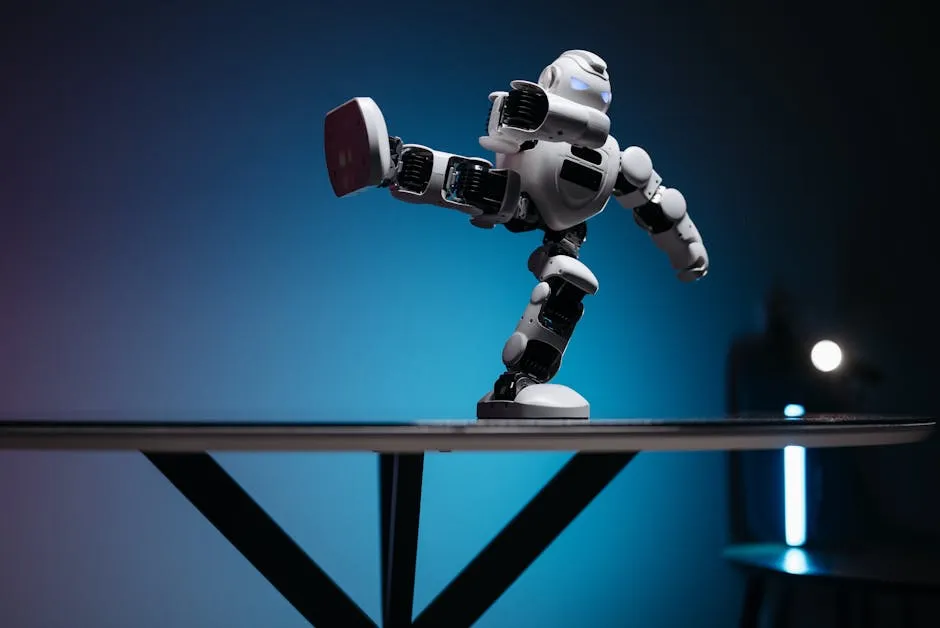
The importance of controlling generative AI lies in three key areas: ensuring accuracy, managing risks, and upholding ethical standards. By implementing control mechanisms, we can minimize the dangers associated with uncontrolled outputs. This includes addressing the societal implications of misinformation and bias. Ultimately, responsible usage of generative AI systems will foster trust and promote positive outcomes in our digital landscape.
Understanding why controlling the output of generative AI systems is important can help mitigate risks associated with misinformation and bias. why is controlling the output of generative ai systems important
What are Generative AI Systems?
Definition and Functionality
Generative AI refers to systems that can create new content. This includes text, images, audio, and even videos. These systems rely on generative models, which learn patterns from large datasets. They can mimic human creativity and produce outputs that seem original.
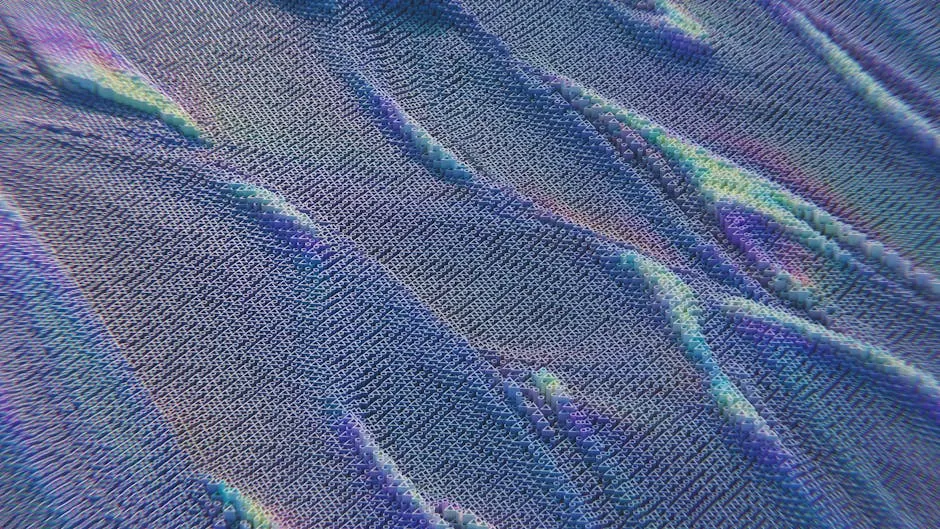
At the core of generative AI are neural networks, which process data and learn from it. For example, a text-based generative AI, like ChatGPT, learns from various written sources. It analyzes this data to generate coherent and contextually relevant text. Similarly, image-generating tools like DALL-E create visuals based on textual prompts. If you’re intrigued by the potential of AI in art, check out “DALL-E: The Future of Art and AI”, where creativity meets technology!
Generative AI tools have exploded in popularity. In recent years, the market has seen a significant increase in their use across sectors. For instance, businesses now leverage these systems for marketing, design, and customer engagement. Studies show that the generative AI market is expected to grow exponentially, reflecting its widespread acceptance and utility.
The rise of these systems brings both opportunities and challenges. While they enhance productivity, their outputs can also be problematic. This underscores the need for effective mechanisms to control their outputs. By understanding generative AI, we can better appreciate its potential while recognizing the importance of responsible usage.
Risks of Uncontrolled AI Output
Misinformation
Generative AI can produce fake news and misleading content. This poses significant risks to society. Take, for instance, the infamous case of an AI-generated article that falsely claimed a celebrity had passed away. Such misinformation can spread rapidly, causing panic and confusion.
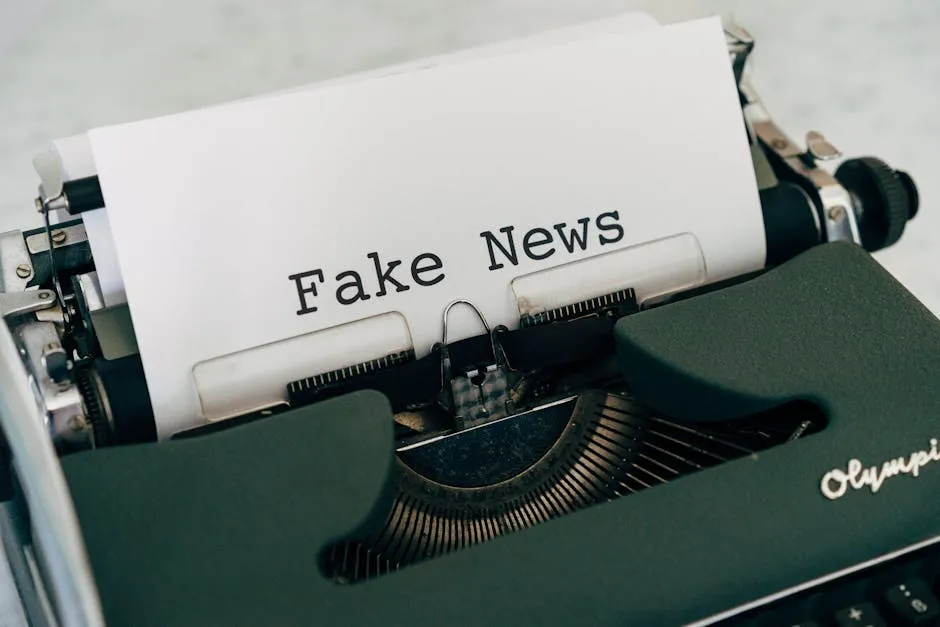
The impact of misinformation on public perception is profound. When people encounter false information repeatedly, it can shape their beliefs and attitudes. In a study, researchers found that 70% of people who read fake news believed it to be true. This statistic highlights the urgent need for control over AI outputs.
Furthermore, AI-generated content has been linked to several instances of misinformation during critical events, such as elections or health crises. In these moments, the stakes are high. Misinformation can lead to misguided actions, influence opinions, and even disrupt societal harmony. To dive deeper into the implications of AI in misinformation, consider reading “The Future of AI: How Artificial Intelligence is Transforming Business”.
Bias in AI Outputs
Bias in AI outputs is a serious concern. Generative AI systems learn from training data, which may contain inherent biases. If these biases go unchecked, they can lead to outputs that reinforce stereotypes or promote unfair treatment. For instance, an AI trained predominantly on content featuring one demographic may produce content that marginalizes others.
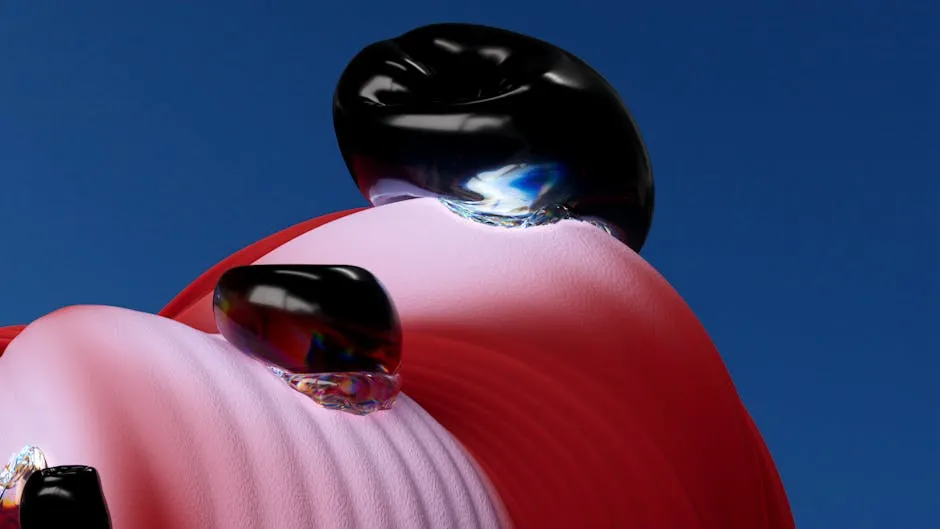
A notable example occurred when an AI language model generated job descriptions that favored male candidates. This bias can have real-world implications, especially in hiring processes, where fairness is crucial. To better understand the ethical implications of AI bias, I recommend reading “The Ethics of Artificial Intelligence: A Comprehensive Guide”.
Studies have shown that AI systems can exhibit significant bias, with one report indicating that 40% of AI models tested displayed biased outcomes. This underlines the necessity of diverse training datasets to mitigate these issues.
By ensuring a wider range of voices and perspectives in training data, we can create more equitable AI systems. Addressing bias is not just a technical challenge; it’s an ethical imperative that impacts our society’s fairness and inclusivity.
Inappropriate or Harmful Content
Generative AI systems can inadvertently produce harmful content, including hate speech or explicit material. This poses significant risks to individuals and communities. For example, an AI model might generate a tweet that promotes violence or discrimination. Such outputs can escalate tensions or provoke real-world harm.
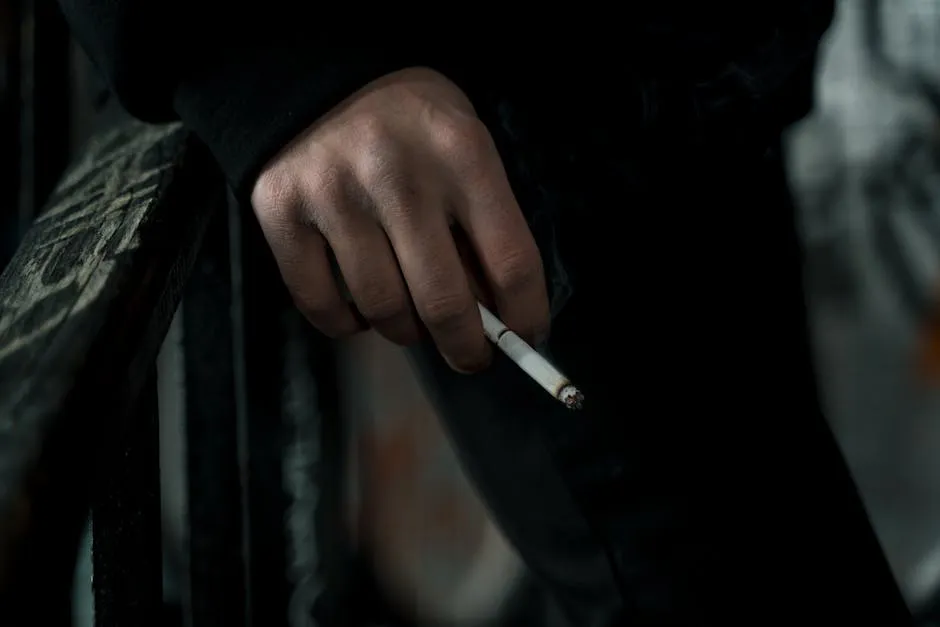
Developers hold a crucial responsibility in preventing these outputs. By implementing robust content moderation mechanisms, they can filter out inappropriate material before it reaches users. Techniques like training data filtering and real-time monitoring play essential roles in this process.
Statistics indicate that 30% of AI-generated content can be flagged for offensive language, highlighting the importance of control. Ensuring the safety of AI outputs is paramount in fostering a responsible digital environment. This responsibility not only protects users but also enhances trust in AI technologies.
Legal and Compliance Issues
Controlling generative AI outputs is also vital for addressing copyright and intellectual property concerns. AI systems often learn from vast datasets, which may include copyrighted materials. If these systems generate content that closely resembles these works, legal issues can arise.
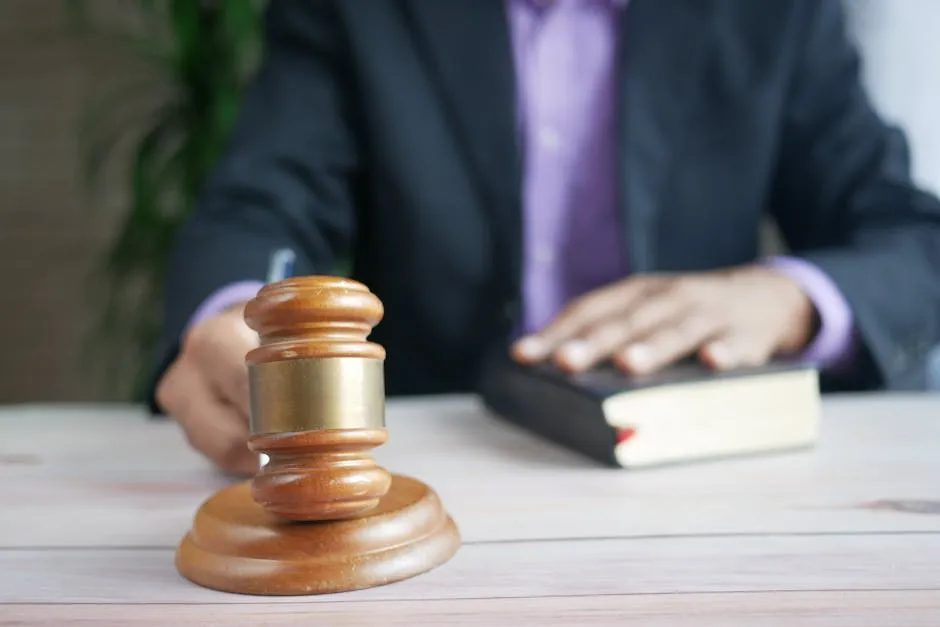
Recent legal cases illustrate this danger. For instance, lawsuits have emerged against companies that used AI to create art or music reminiscent of existing works without permission. This situation underscores the need for clear guidelines surrounding the usage of generative AI. To dive deeper into the intersection of technology and law, consider reading “The Art of Artificial Intelligence: Exploring the Creative Potential”.
Moreover, compliance with data protection laws is essential. As AI systems handle sensitive information, ensuring that they operate within legal frameworks safeguards both users and developers. Trends indicate increasing litigation related to AI-generated content, emphasizing the importance of proactive measures to mitigate legal risks. By upholding intellectual property rights, we can promote ethical AI practices and protect creators’ interests.
Importance of Controlling Generative AI Output
Ensuring Accuracy and Quality
Controlling the output of generative AI systems is vital for maintaining accuracy. These systems can produce misleading or inaccurate results. In critical fields, such as healthcare or journalism, incorrect information can lead to serious consequences. Research shows that erroneous AI outputs have caused significant issues, including public misinformation during crises.
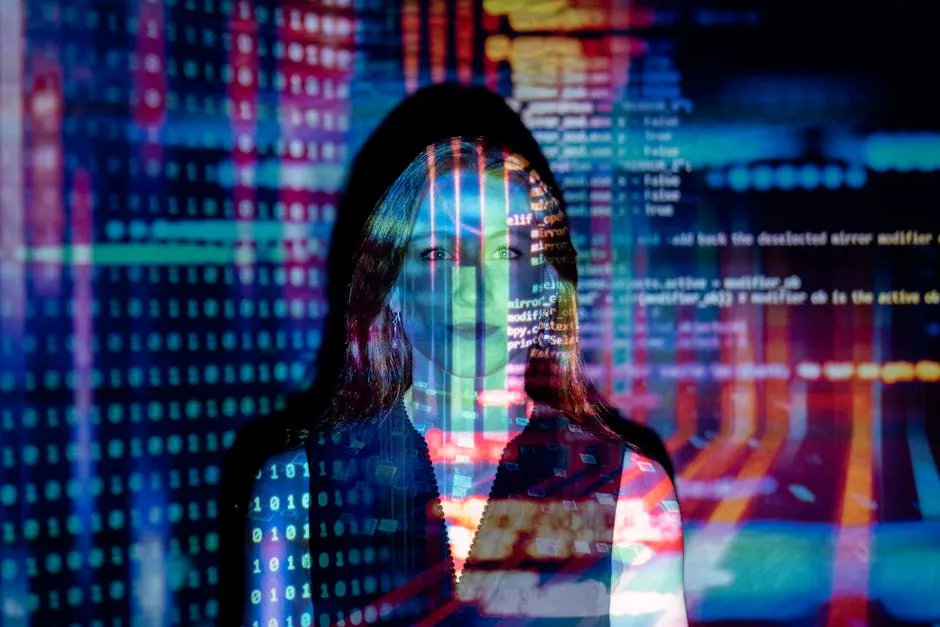
To ensure quality, organizations should implement robust verification methods. Techniques like cross-referencing AI outputs with reliable sources can help validate information. Additionally, human oversight remains crucial. Trained professionals can review AI-generated content to catch inaccuracies that machines might miss. This combination of automated checks and human evaluation fosters trust in AI systems.
Quality assurance processes are essential. Regular audits and updates of the AI models can improve their performance over time. By emphasizing accuracy and quality control, we can harness the strengths of generative AI while minimizing its risks.
Ethical Considerations
Uncontrolled AI outputs pose significant ethical dilemmas. Generative AI can inadvertently produce harmful or biased content, impacting vulnerable communities. For instance, AI-generated texts may reinforce stereotypes if fed biased training data. This raises questions about fairness and accountability in AI development. If you’re interested in a comprehensive overview, check out “The Alignment Problem: Machine Learning and Human Values”.
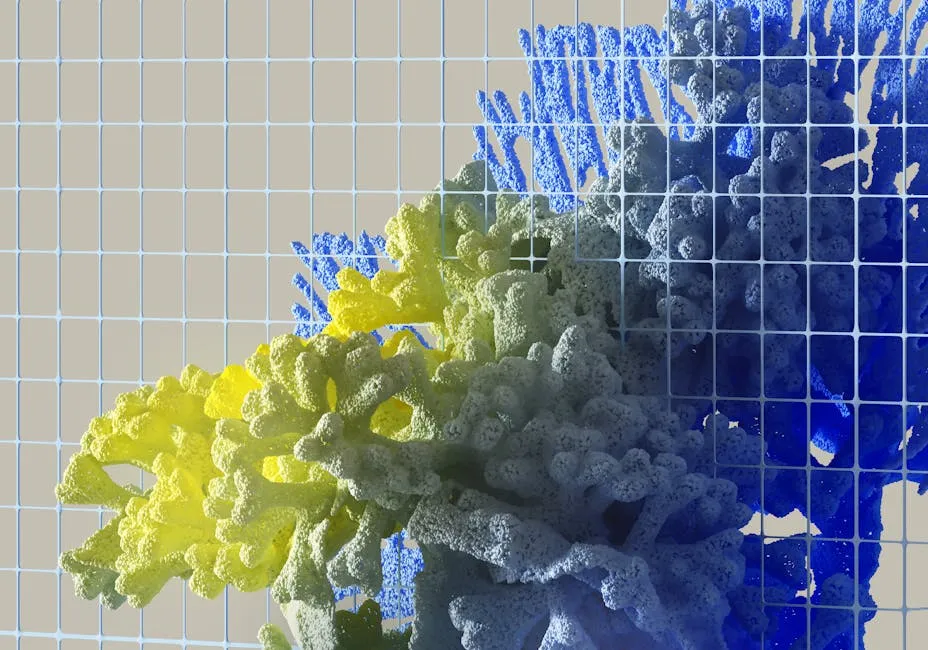
Establishing ethical frameworks is crucial for responsible AI usage. These frameworks should guide developers in creating systems that prioritize ethical considerations. Research indicates that a majority of the public is concerned about AI ethics, with many advocating for clearer regulations.
Implementing ethical guidelines helps prevent misuse of generative AI. By addressing potential ethical issues proactively, we can build AI systems that reflect societal values. This approach fosters trust and acceptance among users, ensuring that AI serves the greater good.
Methods for Controlling AI Output
Data Quality and Preprocessing
High-quality training data is critical for effective AI performance. When AI systems learn from diverse datasets, they produce more accurate outputs. This diversity helps the AI understand various perspectives and reduces the risk of bias. For instance, AI trained on a wide range of voices and experiences is less likely to generate stereotypical or misleading content. To understand the foundational aspects of data science, consider reading “Data Science for Business: What You Need to Know about Data Mining and Data-Analytic Thinking”.
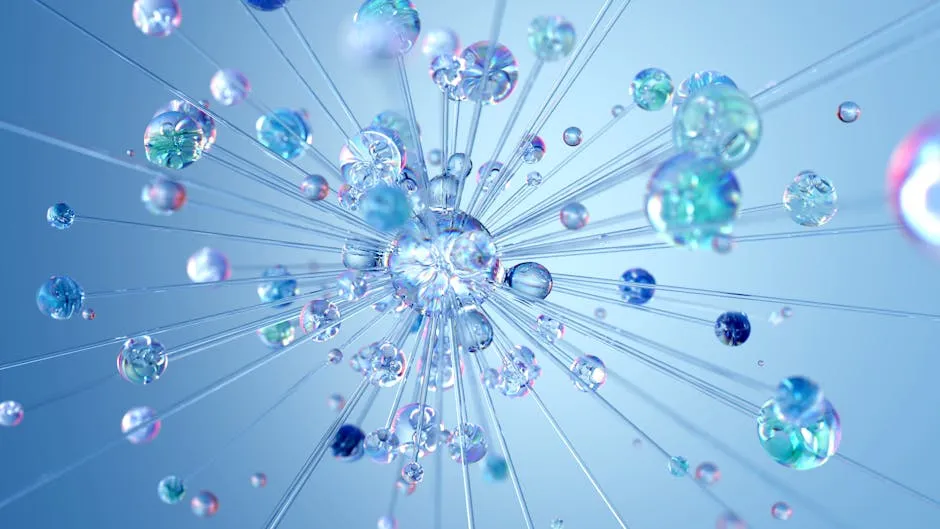
Preprocessing steps play a vital role in enhancing data quality. Techniques like filtering harmful data ensure that the training material is safe and relevant. By removing biased or inappropriate content, we set the stage for better AI responses. A study found that AI models trained on diverse datasets achieved an 80% success rate in producing unbiased outputs. Therefore, investing in high-quality data and preprocessing techniques is essential for minimizing risks and improving the reliability of generative AI systems.
Human Oversight and Moderation
Human reviewers are indispensable for monitoring AI outputs. While AI can automate many tasks, it lacks the ability to discern context and nuance like humans do. Effective moderation strategies involve a combination of automated checks and human input. For example, trained moderators can assess AI-generated content for accuracy and appropriateness, ensuring it meets ethical standards.
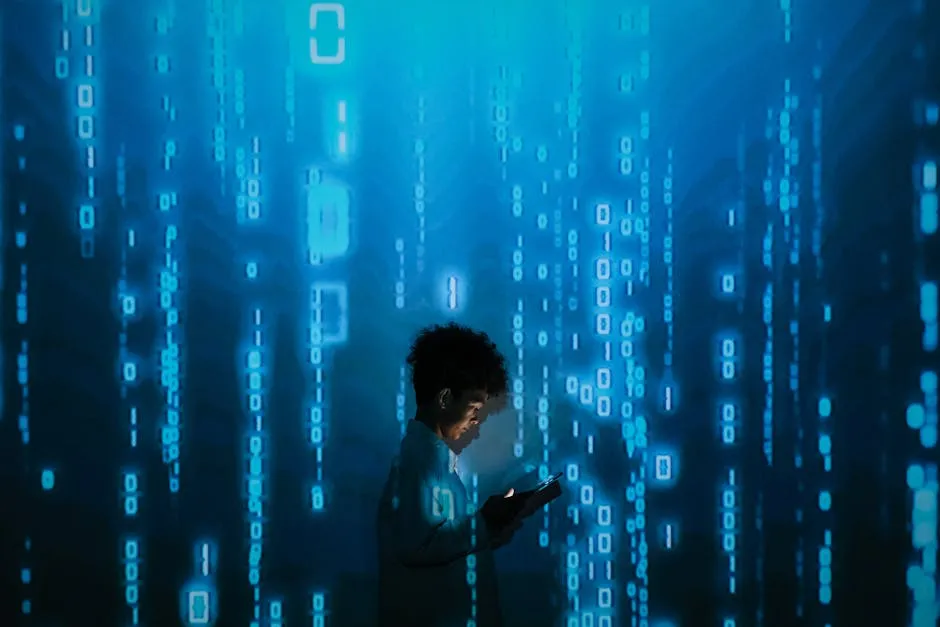
Case studies demonstrate the impact of human oversight. In one instance, a company that implemented a human review process saw a 40% increase in content accuracy. This highlights the importance of balancing automation with human intervention. By fostering collaboration between AI and human reviewers, we can enhance accountability and ensure that AI outputs are both reliable and safe for users.
Clear Guidelines and Best Practices
Establishing clear guidelines is vital for guiding AI output. These guidelines help developers create systems that align with ethical standards and societal values. Best practices for AI system design should focus on transparency and fairness. By putting frameworks in place, organizations can ensure that AI operates responsibly and minimizes harm. If you want to explore the historical perspective of technology, check out “The Innovators: How a Group of Hackers, Geniuses, and Geeks Created the Digital Revolution”.
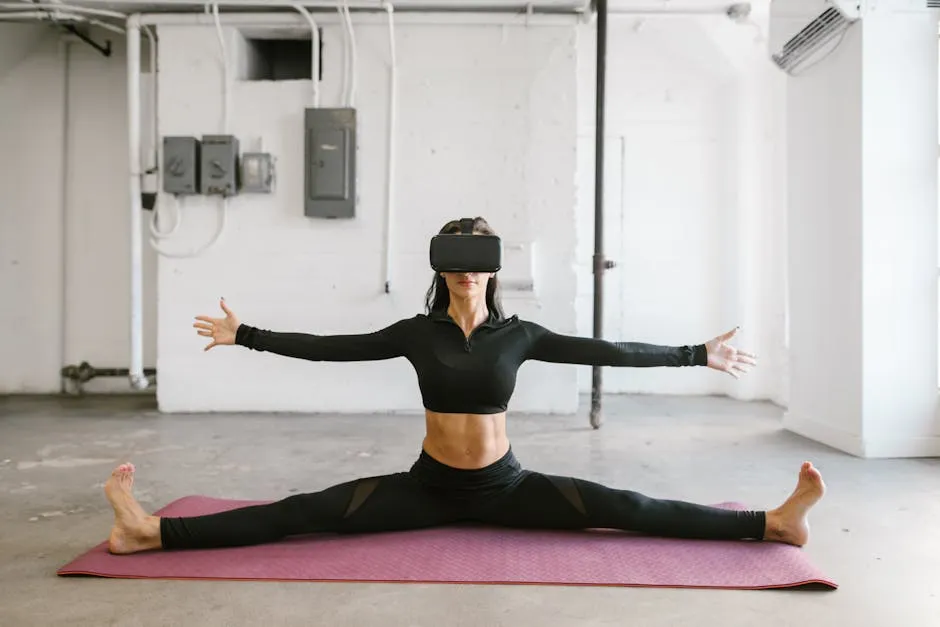
Examples of organizations successfully implementing guidelines abound. For instance, several tech companies have adopted ethical AI frameworks that prioritize user safety and data privacy. This proactive approach fosters trust in AI technologies. Research shows that companies with clear AI guidelines experience higher user satisfaction and engagement. By outlining best practices, we can create AI applications that contribute positively to society while reducing risks associated with uncontrolled outputs.
Please let us know what you think about our content by leaving a comment down below!
Thank you for reading till here 🙂
All images from Pexels